2 Survival models with random effects
Random effects models are a kind of hierarchical model in which the data is assumed to have some sort of hierarchical structure: for instance, individual patients data clustered into families, cities, regions, and so on (Figure 2.1). It is also generally assumed that individuals are homogeneous within a hierarchical unit, heterogeneous between different units; by comparison, fixed effects models do not take into account any hierarchy or heterogeneity in the data. Additionally, the term “fixed effects” traditionally refers to the population-average effects while the term “random effects” refers to subject-specific effects, with the latter generally assumed to be unknown, unobserved variables.
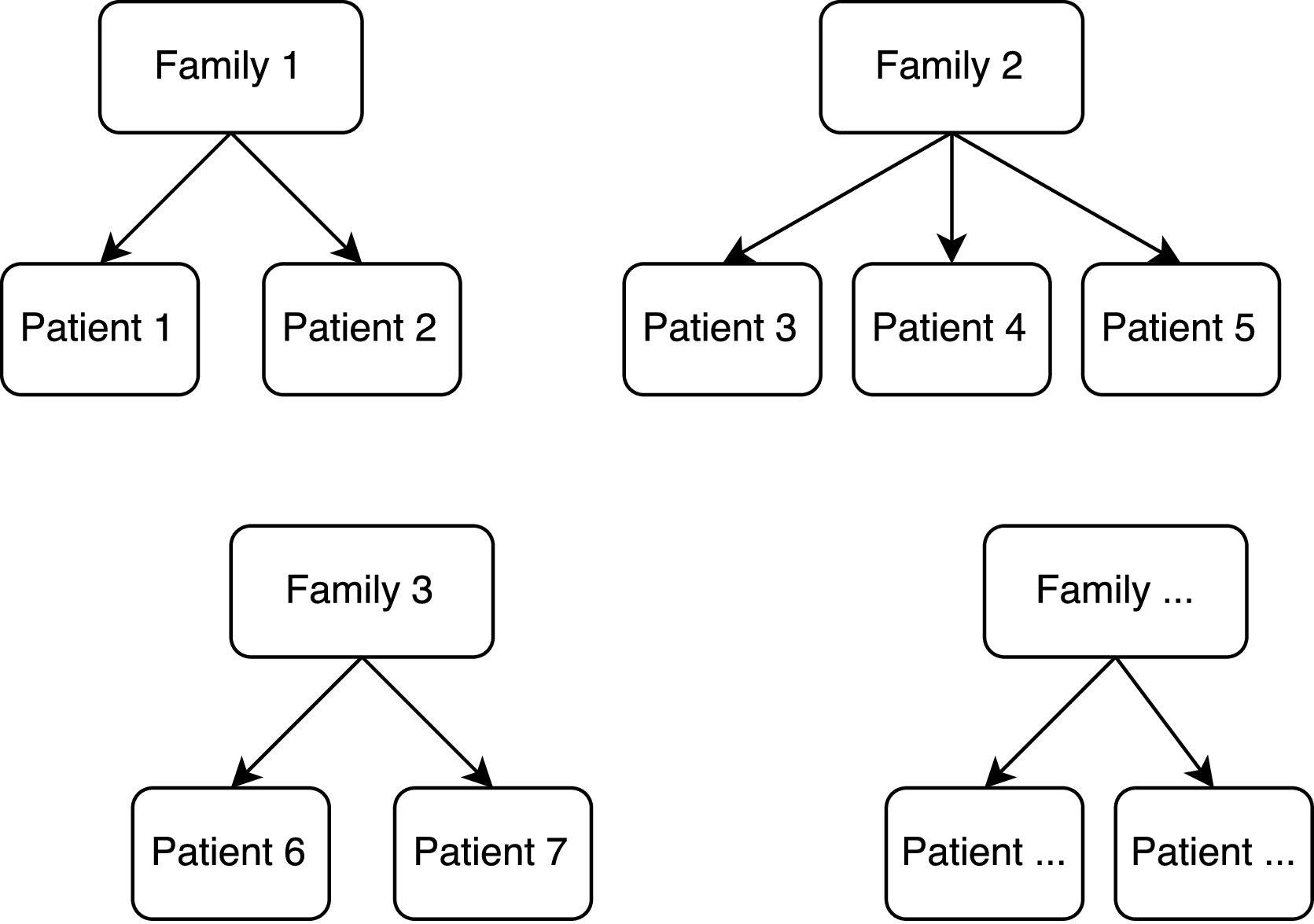
Figure 2.1: Example of clustered data
Random effects models are generally used to analyse hierarchical data with a continuous, normally distributed outcome; such models are referred to as linear mixed-effects models, as they can incorporate both fixed and random effects, and generalise the linear regression model. With data consisting of repeated observations over time, the terms longitudinal data is commonly used. It is possible to encounter hierarchical data originating from a variety of distributions from the exponential family such as the Poisson, Gamma, and Binomial distribution. Linear mixed-effects models can be generalised to include such data, and these models are generally referred to as generalised linear mixed-effects models. Practically speaking, it is the same process of generalising linear models to generalised linear models. Survival data can present a hierarchical structure too; for instance, data could be clustered in geographical areas, institutions, or patients themselves. Meta-analysis of individual-patient data is a common example of survival data (when the outcome is time to event) with some hierarchical structure; another example is given by repeated-events data, such as infections or acute recurrent events, in which the first level of the hierarchical structure consists in the patient. A final example of survival data with biological clusters is given by twin data, in which siblings share some genetic factors. This heterogeneity structure often leads to violation of the implicit assumption that populations are homogeneous: sometimes it is impossible to include all relevant risk factors, or maybe such risk factors are not known at all. The result is unobserved heterogeneity. The simplest survival model with random effects is the univariate frailty model, in which a random effect - named frailty - is included in the model to account for the unobserved heterogeneity. The univariate frailty model can be generalised by allowing the frailty term to be shared between observations belonging to the same cluster of data; the resulting models are named shared frailty model. The frailty term generally acts multiplicatively on the baseline hazard, and it is modelled on the hazard scale; it is possible to alternatively formulate the model in terms of random effects rather than frailties, by including the frailty as an additive term on the log-hazard scale.
I will introduce the univariate frailty model in Section 2.1, and generalise it to allow shared frailty terms in Section 2.2. Finally, I will present the alternative formulation in terms of random effects in Section 2.3. A comprehensive treatment of frailty models in survival analysis is given in Hougaard (2000) and Wienke (2010).
References
Hougaard, Philip. 2000. Analysis of Multivariate Survival Data. Springer New York. doi:10.1007/978-1-4612-1304-8.
Wienke, Andreas. 2010. Frailty Models in Survival Analysis. Chapman & Hall / CRC. doi:10.1201/9781420073911.